Software and AI are the two tools helping scientists navigate the complicated world of cancer biology. By automating tedious tasks and revealing hidden patterns, we experience an acceleration in scientific development that’s bringing us closer to a world where certain cancers become manageable and even curable.
This article will explore how modern wonders like software and AI reshape the fight against cancer.
Data Management and Integration
A big challenge in cancer research is the volume and complexity of data scientists must work with. We’re talking massive amounts of information from various resources —genetic data from tumor samples, patient clinical records, and results from countless experiments and trials. That’s a lot of information to handle, even for a team. That’s where software comes in.
We now have powerful data management tools that can help centralize all this information in one place. Rather than spending hours hunting down data, scientists can quickly find what they’re looking for and spend more time making discoveries.
With software like NovoPath, a robust laboratory information system (LIS), data also becomes easy to share. Cancer research is a collaborative field, with scientists worldwide working together to solve complex problems. The software makes collaboration easy by providing platforms for researchers to share their data and findings easily. By pooling their knowledge and resources, scientists can identify patterns and insights they might have missed if they were working alone.
Genomic Analysis and Personalized Medicine
In the last few years, we’ve learned that cancer is not just a single disease but many different diseases with unique genetic profiles. This has shifted towards personalized medicine, where each patient receives a unique treatment based on their tumor’s genetic makeup.
However, to make personalized medicine possible, scientists need to be able to examine large amounts of genomic data from cancer patients quickly and accurately. LIS software can help streamline the complex sequencing of a tumor’s DNA and find specific mutations driving its growth.
The real power of these tools is in their ability to take this genomic data and use it to recommend targeted therapies that are most likely effective for each patient. For example, if a patient’s tumor has a particular mutation sensitive to a certain drug, AI algorithms can identify that match and recommend a more appropriate treatment.
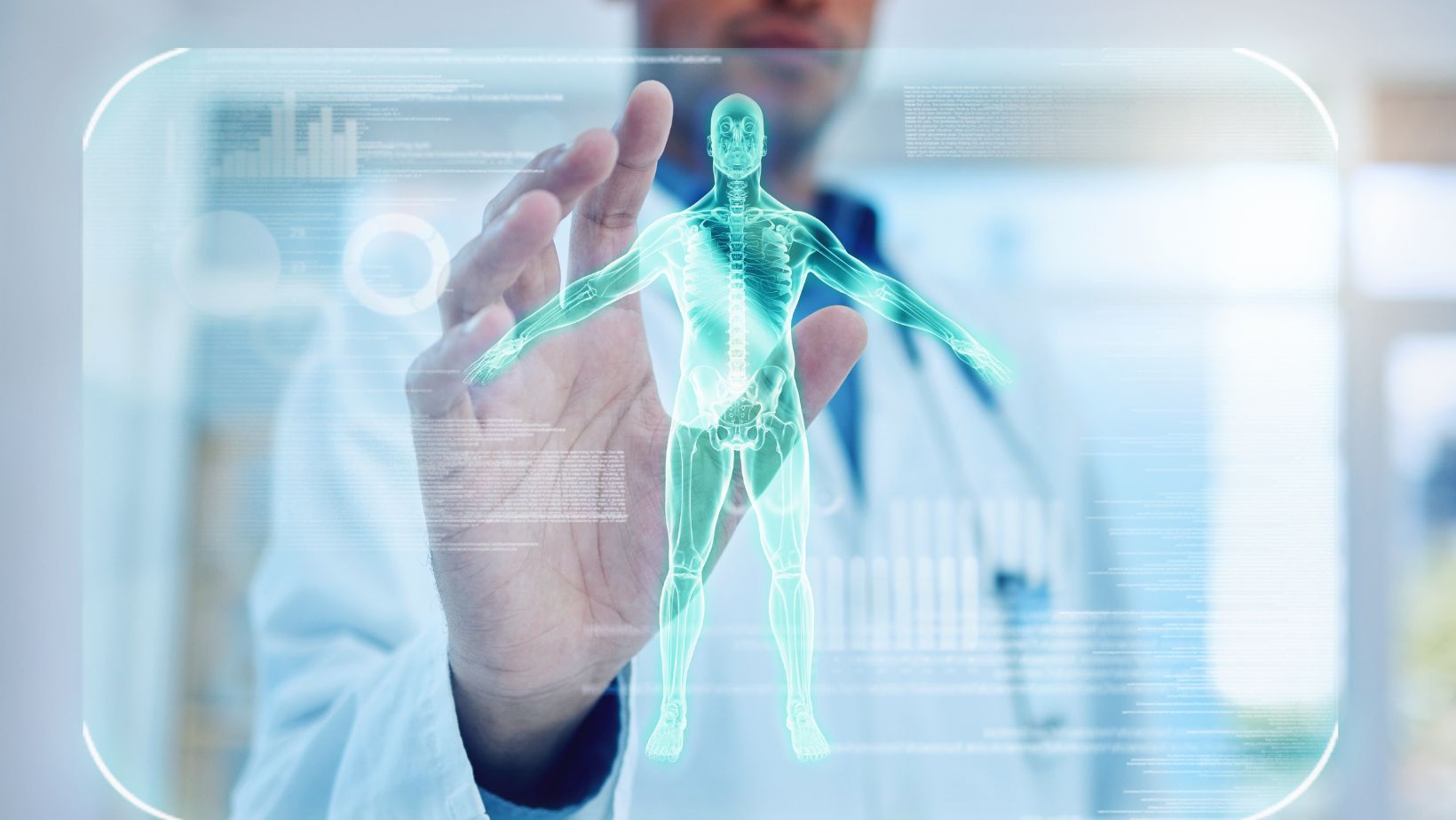
This is a huge step forward over the one-size-fits-all approach to cancer treatment, which was common in the past.
By allowing doctors to precisely target the specific molecular drivers of each patient’s cancer, they can provide more effective, personalized treatments with fewer side effects.
Drug Discovery and Development
It can take years and billions of dollars to develop a new cancer drug, from initial discovery to approval for patient use. But technology can speed up this process. One way is to identify specific proteins or pathways in cancer cells that a drug could target to stop cancer growth. In the past, this was done via trial and error. However, with AI algorithms, we can now analyze large amounts of genetic and molecular data to predict which targets are most likely to be effective.
The next step is designing a drug molecule that binds and interferes with that target. This is where predictive modeling becomes highly useful. Advanced computer models can simulate how different drug molecules interact with their targets, helping researchers optimize the drug’s structure for maximum effectiveness. Companies like CMC Pharma provides critical expertise and technology at this stage, aiding in the streamlining and enhancement of the drug development process.
In the later stages of development, software can streamline processes in experiments and trials, such as collecting and analyzing data. The drug development process becomes more efficient and data-driven, resulting in more effective cancer therapies.
Clinical Trial Management
Clinical trials are essential in developing new cancer treatments but can be time-consuming. A big bottleneck in the process is finding enough patients who meet the specific criteria for a given trial. LIS software can make a big difference here by analyzing a large database of patient records and identifying those who might be eligible.
Once a trial is underway, LIS can collect, monitor, and analyze the test data. This real-time monitoring is very helpful in cancer trials, where patients’ conditions can change rapidly, and unexpected results can happen anytime.
Predictive Modeling and Risk Assessment
AI algorithms can analyze huge data sets of patient information, like genetic profiles, lifestyle factors, and clinical histories, and then identify patterns and risk factors associated with the progression of different types of cancer.
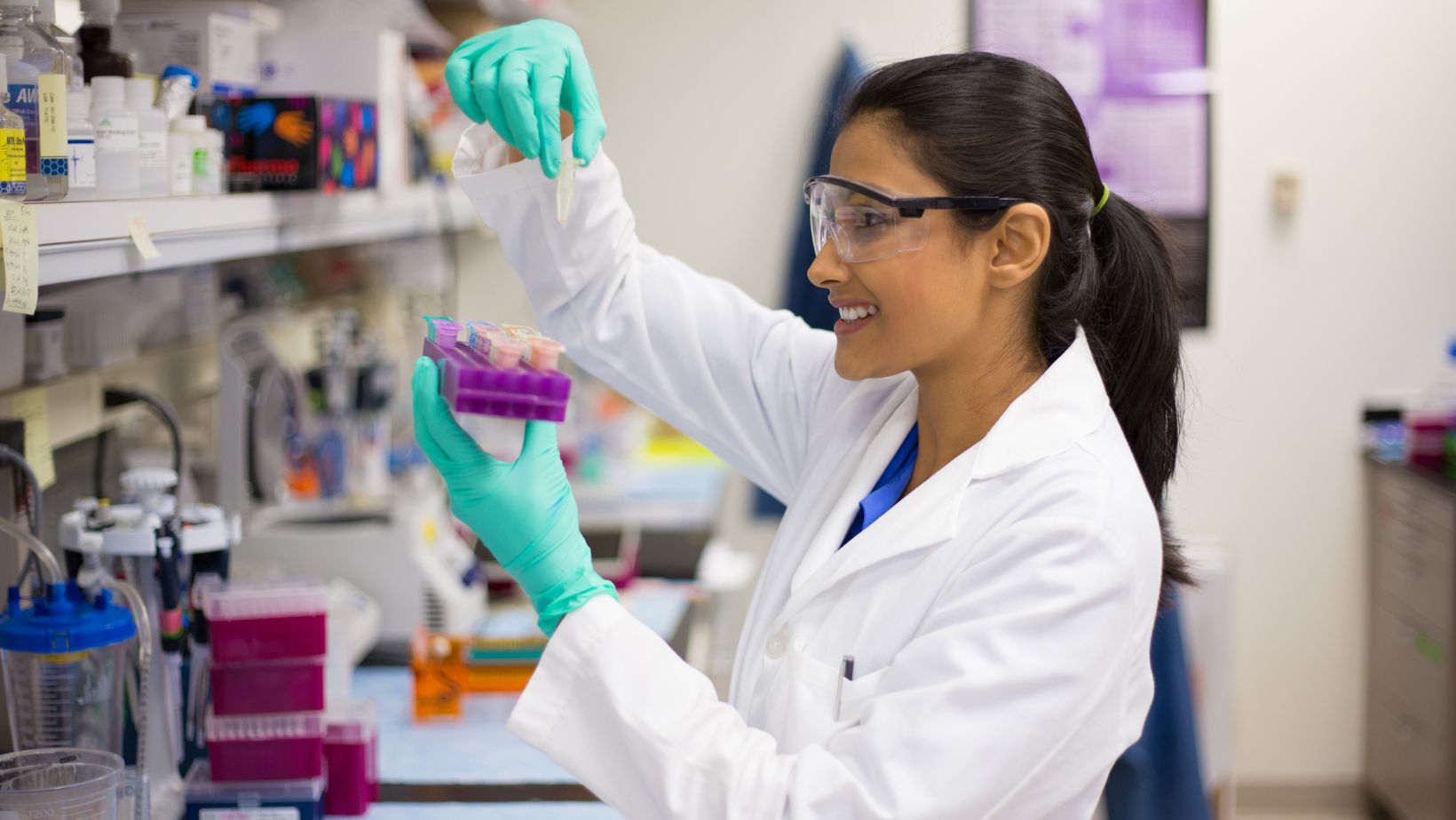
These models can then predict an individual’s likelihood of developing cancer-based on their specific profile, allowing for more targeted prevention and screening strategies.
This early targeted intervention can significantly improve cancer outcomes and save lives. As more data is collected and incorporated into these AI learning models, they will become more accurate at personalizing cancer prevention and care.
Addressing Cancer Research with Technology
By leveraging data and computation, we see new possibilities for understanding, preventing, and treating cancer. These tools not only make research more efficient but also improve outcomes.
Imagine a future where cancer risk can be predicted and treated precisely and treatments tailored to each patient’s unique biology. That is the promise of software and AI. It’s not just about making incremental progress but fundamentally changing the face of cancer research.